A Biologically Plausible, Computationally Efficient Model of the Primary Somatosensory Cortical Column in Mouse
Filed under:
Computational neuroscience
Chao Huang (Laboratory of Neural Circuits and Plasticity, University of Southern California), Andrey Resnik (Laboratory of Neural Circuits and Plasticity, University of Southern California), Tansu Celikel (Laboratory of Neural Circuits and Plasticity, University of Southern California)
To understand how neural circuits perform their functions, it is necessary to quantify the interactions among each individual elements of the circuit, i.e. neurons. Thanks to the advances in high-density microelectrode array recordings and two-photon imaging it is now possible to observe tens to hundreds of neurons simultaneously in action. However, experimental study of every neuron in a functionally relevant circuit is still several decades away. Thus, establishing a biologically plausible and computationally efficient model of neural circuits has an outstanding potential to greatly advance our understanding on the neuronal basis of circuit behavior.
Here we present such a model of the primary somatosensory cortex (S1) of the mouse. The location (i.e. columnar and laminar) and identity (i.e. excitatory vs inhibitory, including subclassification of the major inhibitory subclasses) of the nodes in this topological network of the canonical cortical column is based on cell body reconstructions of the somatosensory cortex using multifluorescence serial confocal microscopy (Huang et al, forthcoming; Fig.1A). The statistics and efficacy of functional connectivity between pairs of cells across and within Layer (L) 4 and L2/3 are modeled after previously published data (e.g.1-7). The mathematical model of individual neurons was based on the quadratic model neuron originally introduced by Izhikevich (8) with the exception that, to free the spike threshold parameter and ensure that spike timing is an emergent property of the network activity, we have derived the spike threshold from the first derivative of the membrane potential during synaptic input after experimentally determining the relationship between the two across membrane states in whole cell recordings in S1.
Simulations showed that, in a network where spiking activity of the presynaptic model neurons are determined according to the probability of single neuron firing in the biological cortical column in vivo, the behavior of the postsynaptic model neurons was statistically comparable to biological neurons recorded in vivo in all paramaters tested. Subthreshold postsynaptic potentials and suprathreshold action potential statistics of the model neurons were comparable to their biological counterparts studied during whisker deflections (Fig.1B-D). These results are discussed in terms of emergent and state-dependent sensory representations in the somatosensory cortex.
Here we present such a model of the primary somatosensory cortex (S1) of the mouse. The location (i.e. columnar and laminar) and identity (i.e. excitatory vs inhibitory, including subclassification of the major inhibitory subclasses) of the nodes in this topological network of the canonical cortical column is based on cell body reconstructions of the somatosensory cortex using multifluorescence serial confocal microscopy (Huang et al, forthcoming; Fig.1A). The statistics and efficacy of functional connectivity between pairs of cells across and within Layer (L) 4 and L2/3 are modeled after previously published data (e.g.1-7). The mathematical model of individual neurons was based on the quadratic model neuron originally introduced by Izhikevich (8) with the exception that, to free the spike threshold parameter and ensure that spike timing is an emergent property of the network activity, we have derived the spike threshold from the first derivative of the membrane potential during synaptic input after experimentally determining the relationship between the two across membrane states in whole cell recordings in S1.
Simulations showed that, in a network where spiking activity of the presynaptic model neurons are determined according to the probability of single neuron firing in the biological cortical column in vivo, the behavior of the postsynaptic model neurons was statistically comparable to biological neurons recorded in vivo in all paramaters tested. Subthreshold postsynaptic potentials and suprathreshold action potential statistics of the model neurons were comparable to their biological counterparts studied during whisker deflections (Fig.1B-D). These results are discussed in terms of emergent and state-dependent sensory representations in the somatosensory cortex.
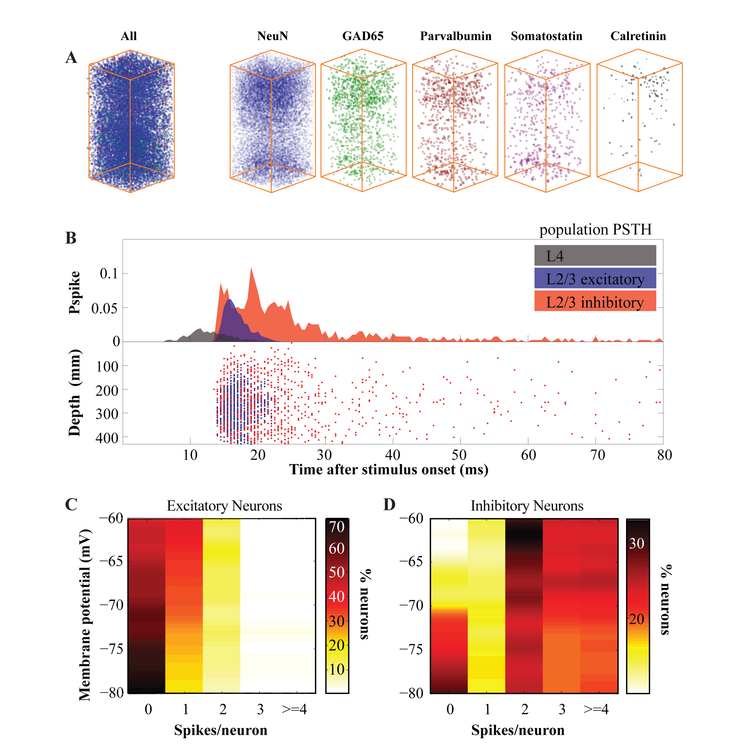
Preferred presentation format:
Poster
Topic:
Computational neuroscience