Estimation method for biophysical properties of insect neurons in the combination of suitable stimulation and multi-compartment simulation with supercomputers
Daisuke Miyamoto (Graduate School of Information Science and Technology, The University of Tokyo ), Tomoki Kazawa (Research Center for Advanced Science and Technology, The University of Tokyo ), Stephan Haupt (Research Center for Advanced Science and Technology, The University of Tokyo ), Masashi Tabuchi (Graduate School of Engineering, The University of Tokyo ), Tomoaki Mori (Graduate School of Information Science and Technology, The University of Tokyo ), Kei Nakatani (Graduate School of Life and Environmental Science, Tsukuba University ), Ryohei Kanzaki (Research Center for Advanced Science and Technology, The University of Tokyo )
We applied this method to projection neurons in antennal lobes of silkmoth (Bombyx mori) as the first model neurons. We obtained morphological tree models containing about 4,000 cylindrical compartments from confocal microscopy images. We assumed the presence of known insect K+, Na+ and Ca2+ channels and used candidate distribution models to estimate the corresponding conductances. Several types of electrical stimuli (current ramps, optionally with added sine waves of different frequencies, and voltage clamp pulses) stimuli, were evaluated for their efficiency in providing multi-compartment models reproducing the electrophysiological data.
Such estimation methods need to run multi-compartment simulations many times. To meet the computational demands of the method, we used the Real Coded Genetics Algorithm (RCGA) for parallel efficiency and MPI/OpenMP hybrid implementations on the K computer which has 640,000 SPARC64VIIIfx CPU cores and on the RIKEN Integrated Cluster of Clusters (RICC).For estimating the position of a high-conductance area in an axon, injecting a current ramp with added sine wave showed good performance even after a small number of generations of the genetic algorithm (Fig. 1).
This could be related to the frequency transmission characteristics of the axon. With 32,768 genes and 200 generations per estimation, we achieved parallel efficiency as high as p=0.99987 comparing the 1,024 cores and 8,192 cores cases (Fig. 2).
We showed here that 1) RCGA is suitable for estimating biophysical properties for realistic multi-compartment models with high efficiency in massively parallel computation and that 2) suitable choice of stimulation paradigms can improve the efficiency of estimation. The interaction between experimental data simulation and parameter estimation can be a useful tool to improve multi-compartment models.
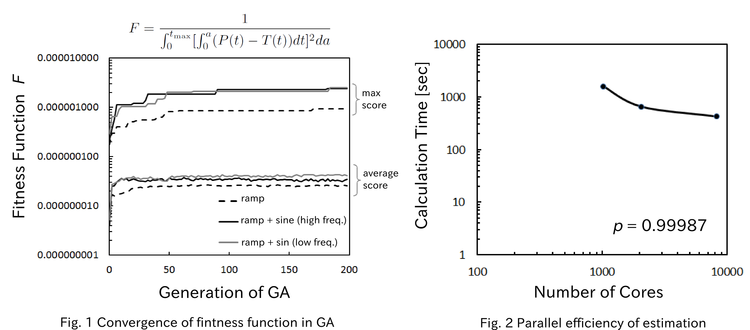