Modeling realistic extracellular recordings of neuronal populations for the purpose of evaluating automatic spike-sorting algorithms
Filed under:
Computational neuroscience
Espen Hagen (Dept. Mathematichal Sciences and Technology, UMB), Torbjørn B Ness (Dept. Mathematichal Sciences and Technology, UMB), Amir Khosrowshahi (Dept. Mathematichal Sciences and Technology, UMB), Felix Franke (Bio Engineering Laboratory, ETH Zürich), Gaute T Einevoll (Dept. Mathematichal Sciences and Technology, UMB)
Simultaneous recordings of thousands of extracellular spikes are now possible, using silicon-based recording devices with large numbers of electrode contacts, such as high-density multi-electrode arrays (MEA) or multi-shank laminar electrodes. With the associated increase of data amounts and complexity in such recordings, manual spike sorting is not viable. Hence, there is a dire need for validated automated spike sorting methods, able to correctly resolve spikes of single neurons, as recently reviewed [1].
Automated spike sorting methods should ideally be validated against test data with known ground truth, where spiking activity of all neurons in the neuronal population is known. Such details of the underlying activity can only to some extent be acquired experimentally. One remedy is model-based simulation of extracellular recordings, as electrode position-dependent spike shapes (Figure 1a) can conveniently be modeled in a biophysically realistic way using a recently released simulation tool, LFPy (http://compneuro.umb.no/LFPy). LFPy implements a forward modeling scheme for extracellular potentials [2] in Python integrated with NEURON [3].
Test data for arbitrary electrode layouts, neuron models, noise content and spike time correlations can be produced at wish, and test data mimicking tetrode and polytrode recordings in cortex and hippocampus with realistic noise features will be presented. Additionally, finite element methods (FEM) are employed to generate test data for cases where significant effects from inhomogeneous extracellular media are present, as in recordings from cell cultures or retinal slice recordings using MEAs.
In order to facilitate usage of benchmark test data for evaluating spike sorting algorithms (Figure 1b), an algorithm evaluation website has been set up on http://www.g-node.org/spike
Acknowledgements:
Supported by the Research Council of Norway (NevroNor, eScience, Notur), NIH (CRCNS) and INCF (G-Node, Norwegian Node).
References:
1. Einevoll GT, Franke F, Hagen E, Pouzat C, Harris KD: Towards reliable spike-train recordings from thousands of neurons with multielectrodes. Curr Opin Neurobiol 2011, 22:1–7. DOI:10.1016/j.conb.2011.10.001
2. Holt GR, Koch C. Electrical interactions via the extracellular potential near cell bodies. J Comp Neurosci 1999, 6:169-184. DOI:10.1023/A:1008832702585
3. Hines ML, Davison AP, Muller E: Neuron and Python. Front Neuroinformatics 2009, 3:1-12. DOI:10.3389/neuro.11.001.2009
Automated spike sorting methods should ideally be validated against test data with known ground truth, where spiking activity of all neurons in the neuronal population is known. Such details of the underlying activity can only to some extent be acquired experimentally. One remedy is model-based simulation of extracellular recordings, as electrode position-dependent spike shapes (Figure 1a) can conveniently be modeled in a biophysically realistic way using a recently released simulation tool, LFPy (http://compneuro.umb.no/LFPy). LFPy implements a forward modeling scheme for extracellular potentials [2] in Python integrated with NEURON [3].
Test data for arbitrary electrode layouts, neuron models, noise content and spike time correlations can be produced at wish, and test data mimicking tetrode and polytrode recordings in cortex and hippocampus with realistic noise features will be presented. Additionally, finite element methods (FEM) are employed to generate test data for cases where significant effects from inhomogeneous extracellular media are present, as in recordings from cell cultures or retinal slice recordings using MEAs.
In order to facilitate usage of benchmark test data for evaluating spike sorting algorithms (Figure 1b), an algorithm evaluation website has been set up on http://www.g-node.org/spike
Acknowledgements:
Supported by the Research Council of Norway (NevroNor, eScience, Notur), NIH (CRCNS) and INCF (G-Node, Norwegian Node).
References:
1. Einevoll GT, Franke F, Hagen E, Pouzat C, Harris KD: Towards reliable spike-train recordings from thousands of neurons with multielectrodes. Curr Opin Neurobiol 2011, 22:1–7. DOI:10.1016/j.conb.2011.10.001
2. Holt GR, Koch C. Electrical interactions via the extracellular potential near cell bodies. J Comp Neurosci 1999, 6:169-184. DOI:10.1023/A:1008832702585
3. Hines ML, Davison AP, Muller E: Neuron and Python. Front Neuroinformatics 2009, 3:1-12. DOI:10.3389/neuro.11.001.2009
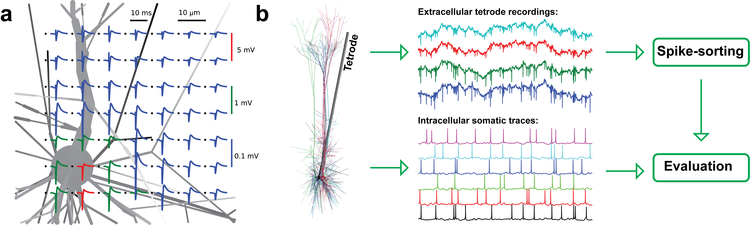
Preferred presentation format:
Poster
Topic:
Computational neuroscience