Sensorimotor Modeling of Speech Production, Speech Perception, and Speech Acquisition
Filed under:
Computational neuroscience
Bernd J. Kroeger (Neurophonetics Group at DPPCD, RWTH Aachen Univ., Germany; School of Computer Science, Tianjin Univ., China ), Cornelia Eckers (Neurophonetics Group at DPPCD, RWTH Aachen University, Germany), Christiane Neuschaefer-Rube (Department of Phoniatrics, Pedaudiology, and Communication Disorders (DPPCD), RWTH Aachen University, Germany)
Our model of speech production, speech perception, and speech acquisition has been implemented and tested by simulating early phases of speech acquisition (i.e. babbling phase and imitation phase) and by performing production and perception tests after learning (Kröger et al. 2009). The detailed structure of the model is given in Fig. 1. A characteristic feature of our approach is that we assume a self-organizing phonetic map which is associated with working memory state maps (distributed neural representations), representing the motor plan, the somatosensory activation pattern (tactile and proprioceptive), and the auditory activation pattern of syllables.
Speech acquisition is simulated in our approach by applying a huge amount of training items to the model. These training items represent stimuli, which are exposed to a newborn and later on to a toddler during the first two years of lifetime. Acquisition starts with “babbling”, i.e. a training phase which is mainly language independent. Here the model generates random motor patterns (motor plan states) and produces appropriate auditory and somatosensory patterns (auditory and somatosensory states). Motor plan and sensory states are exposed to the model nearly simultaneously and thus allow associative learning, i.e. an association of specific motor plan states with corresponding sensory states (Kröger et al. 2009). This learning leads to an adjustment of synaptic weights between neurons of state maps and neurons of the self-organizing phonetic map. Neurons within the phonetic map represent specific sensorimotor states and these states are ordered with respect to phonetic features within this map. This initial sensorimotor babbling training later on allows “imitation training”, because now the model is able to generate motor patterns, if external auditory stimuli are given by an external speaker ("mother"). Imitation training leads to a further ordering of states within the phonetic map and to language-specific speaking skills.
After babbling and imitation training (imitaton of Standard German), the current version of our model has associated motor plan and sensory representations of the 200 most frequent syllables of Standard German and is capable of reproducing and perceiving (identifying) these syllables.
References:
Kröger BJ, Kannampuzha J, Neuschaefer-Rube C (2009) Towards a neurocomputational model of speech production and perception. Speech Communication 51, 793-809
Speech acquisition is simulated in our approach by applying a huge amount of training items to the model. These training items represent stimuli, which are exposed to a newborn and later on to a toddler during the first two years of lifetime. Acquisition starts with “babbling”, i.e. a training phase which is mainly language independent. Here the model generates random motor patterns (motor plan states) and produces appropriate auditory and somatosensory patterns (auditory and somatosensory states). Motor plan and sensory states are exposed to the model nearly simultaneously and thus allow associative learning, i.e. an association of specific motor plan states with corresponding sensory states (Kröger et al. 2009). This learning leads to an adjustment of synaptic weights between neurons of state maps and neurons of the self-organizing phonetic map. Neurons within the phonetic map represent specific sensorimotor states and these states are ordered with respect to phonetic features within this map. This initial sensorimotor babbling training later on allows “imitation training”, because now the model is able to generate motor patterns, if external auditory stimuli are given by an external speaker ("mother"). Imitation training leads to a further ordering of states within the phonetic map and to language-specific speaking skills.
After babbling and imitation training (imitaton of Standard German), the current version of our model has associated motor plan and sensory representations of the 200 most frequent syllables of Standard German and is capable of reproducing and perceiving (identifying) these syllables.
References:
Kröger BJ, Kannampuzha J, Neuschaefer-Rube C (2009) Towards a neurocomputational model of speech production and perception. Speech Communication 51, 793-809
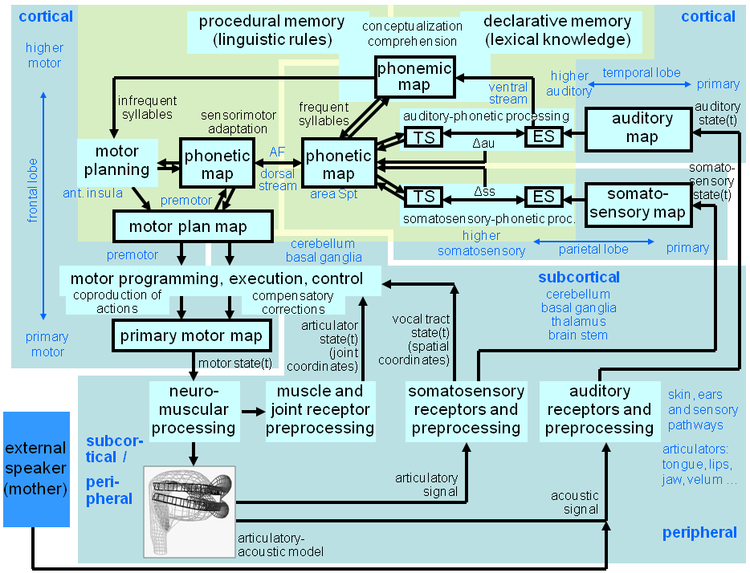
Preferred presentation format:
Poster
Topic:
Computational neuroscience