Sonja Grün
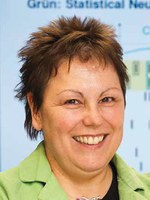
Keynote lecture
Will talk about: Data driven analysis of spatio-temporal cortical interaction
since 2011 Prof. for Theoretical Systems Neurobiology, Faculty I, RWTH Aachen Univ
since 2011 Vice director of Institute for Neuroscience and Medicine (INM-6)
and Leader of Statistical Neuroscience, Forschungszentrum Juelich, Germany
2010 - 2011 Team leader, RIKEN Brain Science Institute, Wako-Shi, Japan
2006 - 2010 Unit leader, RIKEN Brain Science Institute, Wako-Shi, Japan
2004 - 2006 Assitant Prof. (C2) for Theoretical Neuroscience and Neuroinformatics,
Freie Univ Berlin
2002 - 2006 'Forschungsdozentur' for Theoretical Neuroscience and Neuroinformatics
(Stifterverband dt. Wissenschaft), Freie Univ Berlin
2003 Habilitation and Venia Legendi in Neurobiology and Biophysics,
Albert-Ludwigs Univ, Freiburg i. Brsg.
1998 - 2002 Senior fellow, Max-Planck Inst for Brain Reserach, Frankfurt/Main
1996 - 1997 Postdoc, Hebrew Univ, Jerusalem, Israel
1996 Dr. rer. nat., Faculty of Astronomy and Physics, Ruhr-Univ, Bochum
1991 - 1996 Research assistant, Ruhr-Univ Bochum
and Weizmann Inst of Science, Rehovot, Israel
1991 Diploma in physics, Eberhard-Karls Univ, Tuebingen;
Diploma work Max-Planck Inst. for Biological Cybernetics, Tuebingen
1983 - 1991 Study of psychology and physics, Eberhard-Karls Univ, Tuebingen
1980 - 1983 Addtional schooling to university entrance diploma (2. Bildungsweg)
1977 - 1980 Education as Electronician at IBM, Sindelfingen
The mechanisms underlying neuronal information processing and in particular the role of temporal spike coordination are hotly debated. However, the debate is often confounded by an implicit discussion on the use of appropriate analysis methods. To avoid false interpretation of data the analysis of simultaneous spike trains for cooperative neuronal interactions needs to be properly adjusted to the features of experimental data. In particular non-stationarity of the firing of individual neurons in time or across trials, a spike train structure deviating from Poisson, or a co-occurrence of such features in parallel spike trains, are potent generators of false positives. Problems can be avoided by including those features in the null-hypothesis of significance tests. In this context the usage of surrogate data becomes increasingly important, since the complexity of the data typically prevents analytical solutions [1,2]. Thorough testing and calibration of analysis tools is emphasized, also with respect to the impact of potentially erroneous preprocessing stages. The lecture provides an overview of the potential obstacles in the correlation analysis of parallel, also massively parallel, spike data and routes to overcome them [3].
[1] Grün S. Data-driven significance estimation of precise spike correlation. Journal of Neurophysiology, 101, 1126-1140, (2009). (invited review)
[2] Louis S, Gerstein GL, Grün S, Diesmann M. Surrogate spike train generation through dithering in operational time. Frontiers in Computational Neuroscience 4: 127, doi: 10.3389/ fncom.2010.00127 (2010)
[3] Analysis of parallel spike trains. S. Grün and S. Rotter, editors. Springer Series on Computational Neuroscience 106, ISBN 978-1-4419-0377-8 (2010)